Artificial Intelligence (AI) and Machine Learning (ML) are terms that have surged into mainstream discourse over the past decade, shaping industries, revolutionizing technology, and transforming how we interact with the digital world. But despite their ubiquity, confusion often surrounds what these terms actually mean, where they come from, and why they matter.
Defining AI and ML
Artificial Intelligence (AI) refers to the broad field of computer science focused on building machines capable of performing tasks that typically require human intelligence. These tasks include reasoning, problem-solving, understanding language, recognizing patterns, and even decision-making.
AI is not one monolithic technology but a collection of systems and methodologies that allow machines to simulate human-like capabilities. It encompasses everything from simple rule-based systems to complex neural networks.
Machine Learning (ML), on the other hand, is a subfield of AI. ML focuses on the idea that machines can learn from data, identify patterns, and make decisions with minimal human intervention. Rather than being explicitly programmed to perform a task, ML algorithms are “trained” using large datasets to recognize correlations and improve over time.
In simple terms: AI is the broader concept, and ML is one of the most successful approaches for achieving it.
A Brief History of AI and ML
1950s: The Birth of AI The conceptual roots of AI can be traced back to British mathematician and logician Alan Turing. In 1950, he published the landmark paper “Computing Machinery and Intelligence”, where he posed the question: “Can machines think?” This led to the development of the Turing Test, a criterion for determining a machine’s ability to exhibit intelligent behavior.
By the mid-1950s, computer scientists like John McCarthy, Marvin Minsky, and Herbert Simon were developing early AI programs. McCarthy, in fact, coined the term “Artificial Intelligence” in 1956 at the Dartmouth Conference, widely considered the founding moment of AI as a formal field.
1960s–1970s: The First Wave These decades saw optimism about AI’s potential, with projects aiming to create general intelligence. However, limitations in computational power and data access soon caused progress to stall, leading to what is now referred to as the “AI winter”—a period of reduced funding and interest.
1980s–1990s: Emergence of Machine Learning During this time, the AI field began pivoting towards more practical methods. Researchers turned their attention to expert systems and rule-based logic, but it was the rise of machine learning algorithms—especially decision trees and neural networks—that marked a major turning point.
2000s–Present: Data-Driven Intelligence Thanks to exponential increases in data availability, computing power, and storage, ML began to flourish. Techniques such as deep learning (a more complex form of ML using multi-layered neural networks) made dramatic advances in speech recognition, image classification, and natural language processing. This is the era of AI applications like Siri, ChatGPT, self-driving cars, and medical diagnostic tools.
The Advantages of AI and ML
AI and ML offer numerous advantages, many of which are transforming how we live, work, and solve complex problems:
- Automation of Repetitive Tasks: AI can handle tasks like data entry, customer service, and inventory management, freeing up human workers for more strategic roles.
- Enhanced Decision-Making: ML models can process and analyze vast amounts of data faster than humans, providing insights that aid in decision-making in fields like finance, healthcare, and marketing.
- Personalization: From streaming services to online shopping, AI tailors experiences based on user behavior, preferences, and patterns.
- Predictive Capabilities: ML can forecast trends, from disease outbreaks to equipment failures, allowing organizations to proactively respond.
- Medical Advancements: AI is helping diagnose diseases earlier and more accurately, while ML models analyze genetic data to tailor treatments for individual patients.
- Accessibility and Inclusion: AI-powered tools like real-time language translation, voice-to-text, and smart assistants enhance accessibility for people with disabilities.
While AI and ML carry immense promise, they also present ethical and societal challenges—ranging from algorithmic bias to surveillance concerns and job displacement. Addressing these requires a balanced, transparent approach that centers human well-being, equity, and accountability.
In the evolving landscape of technology, understanding AI and ML is no longer optional—it’s essential. These technologies are not just shaping our future; they’re shaping our present.
Photo by Brian Kostiuk on Unsplash
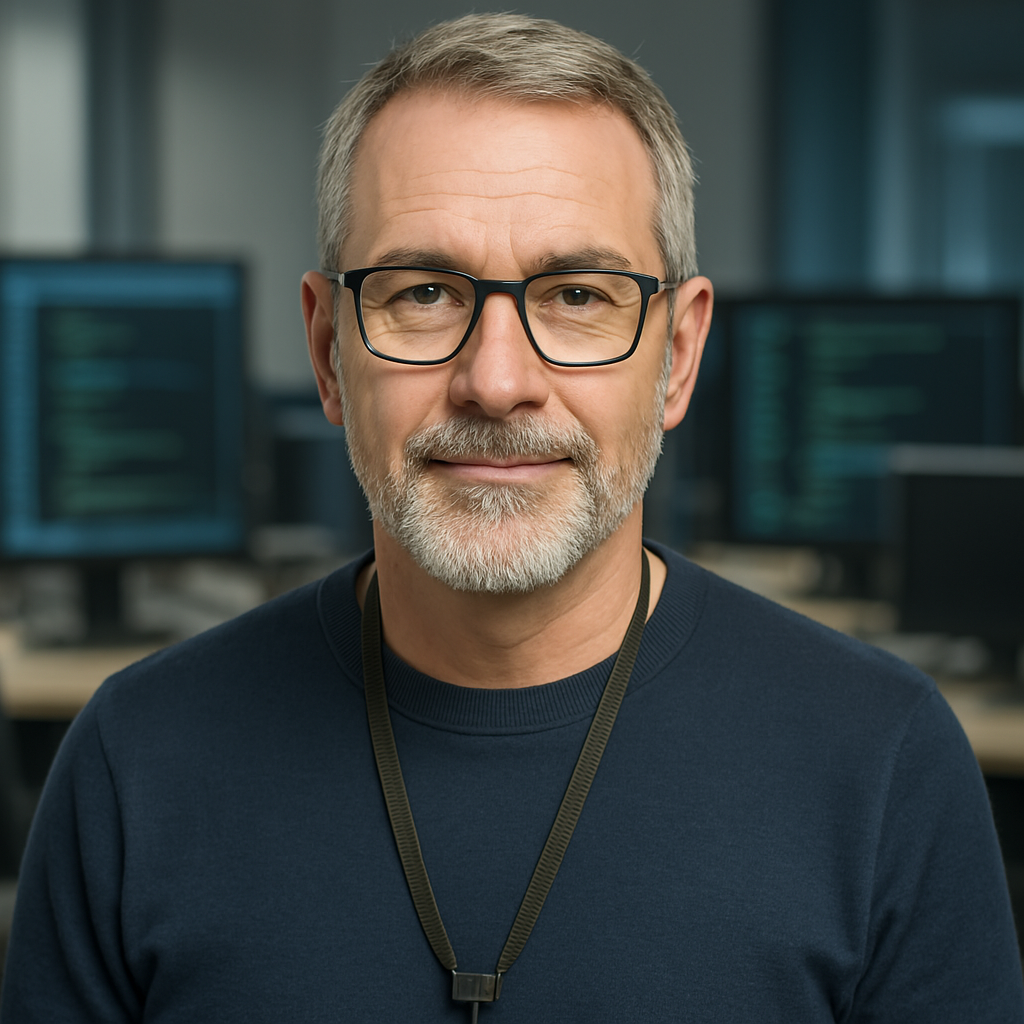
Mark Stevens is a veteran IT Systems Architect with over two decades of hands-on experience in both legacy and modern tech environments. From mainframes and Novell networks to cloud migrations and cybersecurity, Mark has seen it all. When he’s not solving complex IT puzzles, he’s sharing insights on how old-school tech foundations still shape today’s digital world.